Smart Ways to Understand Degrees of Freedom in 2025
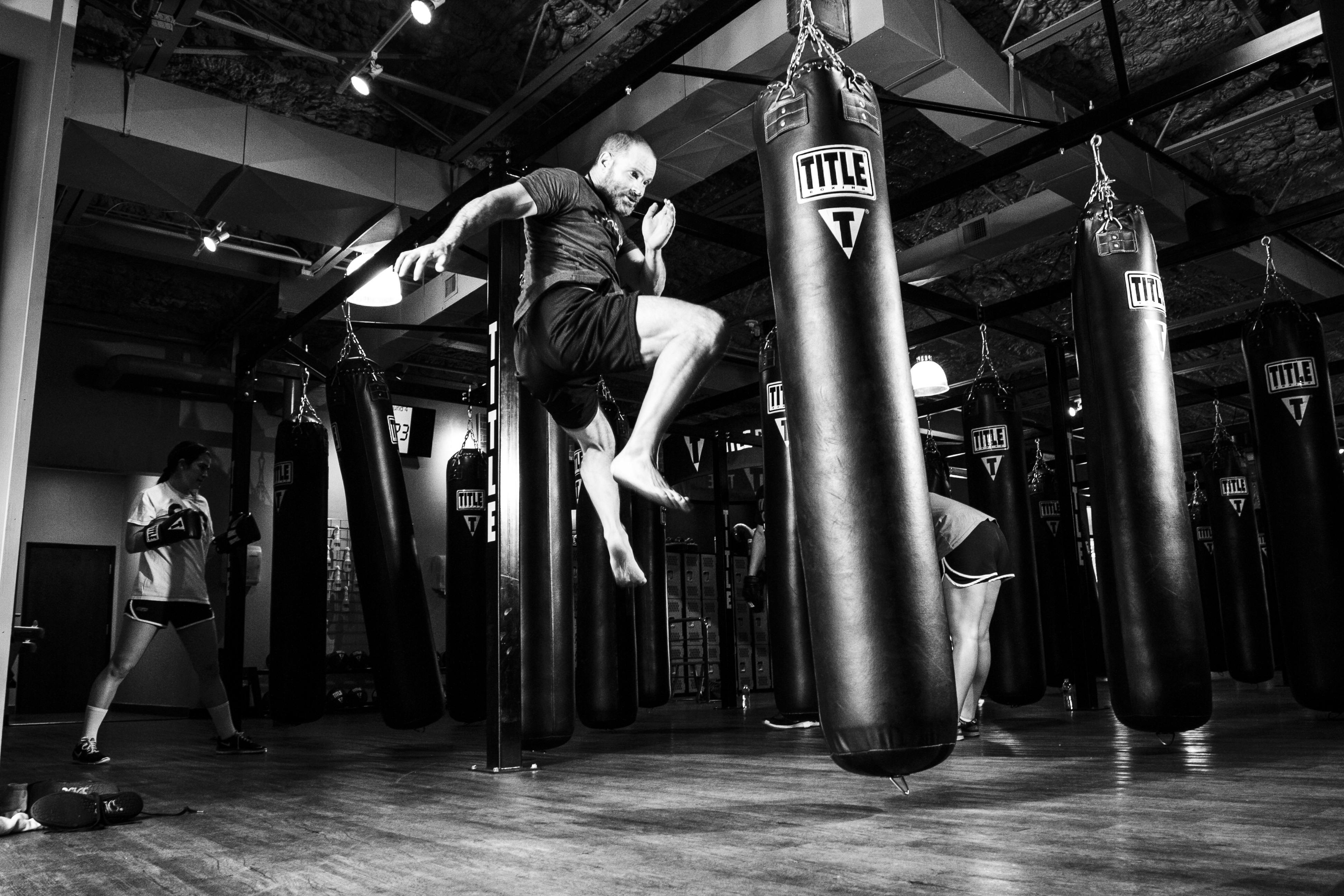
Effective Ways to Calculate Degrees of Freedom: A Practical Guide for 2025
Understanding Degrees of Freedom in Statistical Analysis
Degrees of freedom (df) are crucial in statistical analysis, representing the number of independent values in a calculation. They are essential in various statistical tests, including hypothesis testing, ANOVA, and regression analysis. Understanding df is vital for ensuring accurate results when assessing statistical significance. Moreover, comprehending the various ways to calculate degrees of freedom can enrich your data analysis process and deliver better inferential statistics. In this section, we will explore the fundamental concepts surrounding degrees of freedom, emphasizing their role in statistical methodologies.
Types of Degrees of Freedom
Degrees of freedom vary depending on the statistical test and research design used. Common types include those associated with t-tests, ANOVA, chi-square tests, and regression analysis. For a **t-test**, the degrees of freedom can typically be calculated as the total number of subjects minus one for independent samples. In **ANOVA**, there are different degrees of freedom for both the treatment and error terms, requiring careful consideration of sample sizes and designs. Meanwhile, the **chi-square test** has df calculated based on the number of categories minus one. Understanding these variations helps researchers accurately determine statistically significant outcomes in studies involving dependent and independent variables.
The Degrees of Freedom Formula
The formula for calculating degrees of freedom varies based on the context or statistical test in use. Typically, for a simple one-sample design, the degrees of freedom can be represented by the formula: df = n - 1, where n is the sample size. In the case of two-sample t-tests, the formula becomes more complex, needing the total sample sizes of both groups taken into account. For **ANOVA** designs, the calculation incorporates the number of levels and groups. To ensure accuracy, researchers must adhere to the specific degrees of freedom formula pertinent to their experimental design and statistical test.
The Importance of Degrees of Freedom in Hypothesis Testing
In hypothesis testing, degrees of freedom play a pivotal role in determining the critical values of statistical tests. They influence the shape of the sampling distribution used to estimate p-values, reflecting how confident a researcher can be in rejecting or accepting the null hypothesis. An accurate assessment of **statistical significance** necessitates a proper calculation of df, as it directly impacts the boundaries for determining whether results are due to chance or represent true effects in the population. This consideration is crucial, especially in fields that rely heavily on statistical methodologies for data interpretation and conclusion formation.
Calculating Degrees of Freedom in Different Statistical Tests
Calculating degrees of freedom varies across different statistical tests, each with unique formulas and considerations that apply to their specific research methodologies. Knowledge of these distinctions is vital for accurate statistical inference, ultimately informing research decisions based on solid methodological foundations. In this section, we will dissect how to calculate degrees of freedom in commonly used tests, such as t-tests, ANOVA, and regression analysis.
Degrees of Freedom in T-Tests
In t-tests, which are commonly used to compare the means of two groups, calculating degrees of freedom is straightforward yet essential. For an independent t-test, degrees of freedom are calculated as the sum of both group sizes minus two, represented by the formula: df = n1 + n2 - 2. This calculation enables researchers to determine critical t-values from statistical tables effectively. Understanding this relationship assists in properly assessing whether significant differences exist within sample statistics, reinforcing the conclusions drawn in a study.
Degrees of Freedom in ANOVA
For **ANOVA** (Analysis of Variance), calculating degrees of freedom involves a bit more complexity due to the multi-group comparisons. In a one-way ANOVA, the degrees of freedom for treatment is represented as k - 1 (where k is the number of groups), and for error, it is calculated as N - k, with N being the total number of observations. In **two-way ANOVA**, the calculation involves interactions between independent variables, necessitating consideration of interaction terms. Understanding these calculations allows researchers to assess variance effectively and determine if significant differences exist among group means.
Degrees of Freedom in Regression Analysis
In regression analysis, the calculation of degrees of freedom is essential for testing model fit and significance levels. For a simple linear regression, the degrees of freedom for the model is equal to k (the number of predictors), while the error degrees of freedom is calculated as N - k - 1. This distinction is crucial when analyzing the significance of regression coefficients and the overall model performance. By accurately accounting for degrees of freedom, researchers can better understand the relationship between independent and dependent variables and make more informed decisions in statistical inference.
Common Mistakes in Calculating Degrees of Freedom
Despite its importance, researchers often make mistakes when calculating degrees of freedom, which can lead to incorrect conclusions or misinterpretations of data. Being aware of potential pitfalls can improve the reliability of statistical results and enhance the integrity of research findings. In this section, we will discuss common mistakes made during calculations and provide strategies to avoid them.
Overlooking Sample Sizes
One common error in calculating degrees of freedom is overlooking the actual sample sizes involved in the study. Ensuring the correct sample sizes are used throughout the calculations is crucial, particularly in t-tests and ANOVA. Skipping this step could result in underestimating or overestimating degrees of freedom, leading to flawed statistical significance conclusions. Always verify sample sizes before proceeding with any statistical tests.
Improper Test Selection
Another common mistake is selecting the wrong type of statistical test for the data at hand, specifically regarding the assumptions of parametric versus non-parametric tests. Different tests come with unique requirements for calculating degrees of freedom. For instance, using a t-test on non-normally distributed data violates the assumptions of the test, resulting in inaccurate degrees of freedom. Employing the proper statistical testing aligned with data distribution ensures valid results and appropriate conclusions.
Neglecting Interaction Effects in ANOVA
In ANOVA designs, neglecting to account for interaction effects can significantly impact the degrees of freedom calculations. For two-way ANOVA, understanding how different factors interact can change how researchers interpret results. Ignoring these interaction effects could mislead researchers into drawing incorrect inferences about the significance of their findings. It is vital to consider all necessary factors, including their interactions, to avoid errors in determining degrees of freedom.
Key Takeaways
- Degrees of freedom are essential in statistical analysis and influence hypothesis testing outcomes.
- Accuracy in calculating degrees of freedom varies across different statistical tests such as t-tests, ANOVA, and regression analysis.
- Avoid common mistakes related to sample size, test selection, and interaction effects to ensure reliable results.
- Understanding the degrees of freedom formula and its application is critical for valid statistical inference.
- Proper assessment of statistical models guides researchers in making informed decisions based on data distribution.
FAQ
1. What is the significance of degrees of freedom in statistical analysis?
Degrees of freedom are significant as they determine how many independent values can vary in a statistical calculation. In hypothesis testing and when computing the significance of statistical tests, accurate degrees of freedom calculations ensure valid results and correct conclusions in inferential statistics.
2. How do degrees of freedom influence hypothesis testing?
In hypothesis testing, degrees of freedom influence the critical values derived from statistical distributions, directly impacting the determination of p-values. Ensuring the correct calculation of degrees of freedom is essential for accurately assessing the validity and significance of research findings.
3. What are common errors to avoid when calculating degrees of freedom?
Common errors include overlooking sample sizes, using the wrong statistical tests, and neglecting interaction effects in complex designs like ANOVA. Understanding these common pitfalls can enhance the accuracy and reliability of statistical analyses.
4. Can degrees of freedom be negative?
No, degrees of freedom cannot be negative. They represent independent values available for estimation in a statistical analysis. A proper understanding and calculation of how to derive df in various tests ensure that it remains non-negative.
5. What resources can I use to learn more about calculating degrees of freedom?
Various online platforms, textbooks, and statistical software tutorials are excellent resources. Also, academic articles focusing on research methodologies often provide in-depth examples and explanations for calculating degrees of freedom in various contexts.